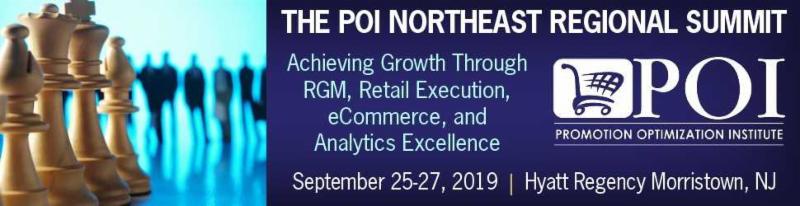
Retail applications
Data utilization starts traditionally through descriptive analytics where we analyze the data to find out what happened. This is the traditional predictive analytics described above. Some vendors are putting a fancy voice-enabled query engine on the front end of this capability and calling it AI/ML, but what you really have is a voice-enabled dashboard running descriptive data queries or some type of search. With ML, once the data has been structured in a descriptive format, it can then be further evaluated with trends being identified and identifying why certain outcomes occurred. Machine learning as an extension of our more traditional predictive analytics looks at those trends and relationships, eventually predicting what will happen with higher accuracy over time.
Retail advantage can be gained by combining our traditional predictive analytics based on historical data with ML which is based on pattern recognition.
Filtering for the preferred minimum or maximum inventory level will provide insight into the average units sold during a specified time frame. We then calculate inventory days of supply that we have on hand which in turn provides a predictive indicator that will identify trends and through management by exception (MBE) we can then alert the appropriate individuals when action is needed.
Items generating higher volume sales analyzed at a store-by-store level over time can uncover trends in consumer behavior and preferences that will provide insights on what items to carry and at what price points. These types of predictions lead to better decision making in ordering, stocking and other factors affecting the company’s bottom line.
Comparing sales and inventory for similar periods of time by geographic region including influencing factors such as competitor brand actions can help to determine adjustments to orders and inventory levels. Further enhance order upside…Learn more click here.
Industry News
Hyatt Morristown, Morristown, NJ
Source: (Forbes June 27, 2019)
Leave a Reply